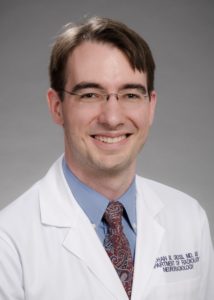
Nathan Cross, MD, MS
Dr. Nathan Cross is an Assistant Professor and Associate Medical Director of Informatics in Neuroradiology at the University of Washington Department of Radiology.
Dr. Cross leads the CLEAR Center’s Image Processing Team (IPG). He has a long history of work in imaging informatics and image processing with a background in engineering and computer science. Dr. Cross is especially interested in in the newest areas of machine learning to the clinical problem of finding fractures in his daily clinical neuroradiology practice. To that end he has assembled a team of expert annotators who then trained through consensus labeling of radiographs to achieve an inter-rater agreement Kappa score of ≥0.6 using the modified Algorithm Based Qualitative criteria. To date, the IPG Team has consensus labelled 300 radiographs containing over 2000 individual vertebral bodies. In order to reduce the burden of manual annotation of images including time, labor, and cost Dr. Cross’s team utilized machine learning to design and implement a Dicom image annotation program. This program is easy to learn, efficient and allows annotators to expeditiously annotate large sets of DICOM images.
Recently, Dr. Cross received an award from AUR GE-Radiology Research Academic Fellowship to conduct research in Clinical Translation of Machine Learning-based Opportunistic Screening for Imaging Evidence of Osteoporosis. This 2-year fellowship provides Dr Cross with 50% research time to develop methodology to analyze radiographs for evidence of osteoporosis and osteoporotic compression fractures and then automatically generate relevant data to guide the ordering physician in the next steps of the patient’s workup such as bone density testing or clinical evaluation for osteoporosis. This could ultimately lead to improved osteoporosis treatment and prevention of future fractures and disability.
Of the fellowship, Dr. Cross says “The GE-AUR Radiology Research Academic Fellowship (GERRAF) is funding my project Clinical Translation of Machine Learning-based Opportunistic Screening for Imaging Evidence of Osteoporosis which will use deep learning to automatically classify osteoporotic compression fractures based on imaging data and begin to incorporate readily available clinical data. A template for presenting these results to practitioners will be created based on interaction with multiple providers throughout the UW caring for those with osteoporosis”.